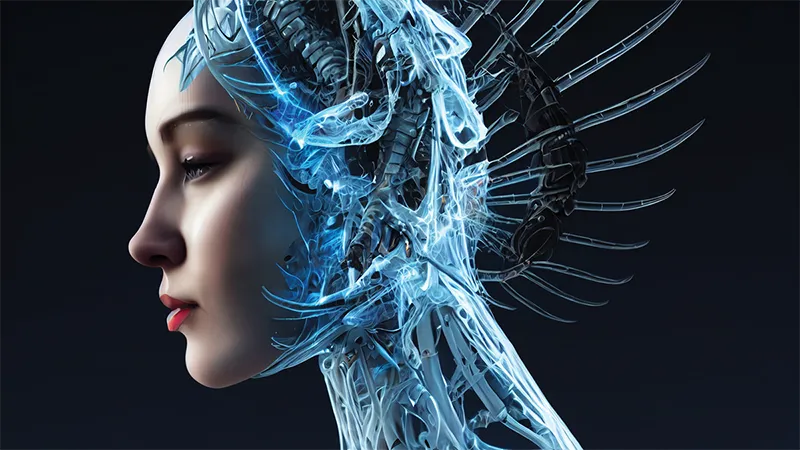
What is Generative AI?
What is Generative AI?
Are you curious about Generative AI and its capabilities to create new content? Look no further, as Cream Digital Ai is here to provide a free Ai consultation for you! As a leading AI developer, we specialize in customized solutions, including our advanced Ai phone agents that can handle various tasks like marketing, sales, customer support, and operations. Read on to learn more about Generative AI and how it can enhance your business.
Generative AI Definition and Differences
Generative AI, short for Generative Adversarial Networks (GANs), is a powerful category of artificial intelligence that has surged to the forefront of the technology landscape. Its capabilities represent a significant leap in the field of machine learning, particularly in the domain of creating new content from existing data. Unlike traditional AI models, which are predominantly used for making predictions or performing tasks based on existing information, generative AI excels in the generation of new and original content through pattern creation and data synthesis. The primary distinction between conventional AI and generative AI lies in their respective capacities. While the former is proficient in recognizing patterns and making decisions based on labeled data, the latter is capable of learning patterns and structures from training data to produce new, previously unseen data, including images, text, and more.
The innovative potential of generative AI extends to a wide array of applications, from enhancing business processes and customer experiences to boosting productivity and efficiency across various industries. Its ability to create new and meaningful content represents a paradigm shift in the way AI is leveraged, opening up opportunities for the development of cutting-edge solutions and the transformation of digital experiences. The adoption of generative AI can lead to improved agility in application development, with the capacity to accelerate the creation of novel applications using platforms such as AWS and the Now Platform. Moreover, the deployment of generative AI has the potential to revolutionize the learning and development sector, as seen in platforms like Udemy, where the technology can be utilized to offer enriched and personalized learning experiences to a global community of learners.
Generative AI Creates New Content
At the core of Generative AI's value proposition is its unparalleled ability to create new and original content from learned data, thereby transcending the limitations of traditional AI models. By harnessing the power of advanced machine learning and deep learning techniques, generative AI models can analyze and understand complex patterns within the training data, ultimately producing new and authentic content that aligns with the learned characteristics and features. This transformative capacity for content generation empowers businesses and individuals to access a wealth of novel possibilities, ranging from the creation of bespoke multimedia content to the development of innovative applications that drive value and engagement.
Empowering AI agents with the capabilities of generative AI opens up new frontiers in customer service, as these advanced systems are capable of delivering more personalized and contextually relevant responses to user prompts and requests. Whether in the form of text-based interactions, voice interfaces, or visual content generation, the integration of generative AI into customer service platforms can significantly elevate the overall customer experience by providing tailored and adaptive solutions that closely align with individual needs and preferences. As a result, the deployment of generative AI in customer service has the potential to not only improve the efficiency of service delivery but also foster stronger and more meaningful connections between businesses and their customers.
Other AI Predicts or Performs Tasks
Conversely, traditional AI models are predominantly oriented towards predicting outcomes or performing specific tasks based on the input data and the established parameters of the model. These models, which encompass a wide range of machine learning algorithms and techniques, are instrumental in various applications, including predictive analytics, decision support systems, and process automation. By leveraging historical and labeled data, traditional AI can make informed predictions and decisions, thereby contributing to enhanced operational efficiency and performance optimization across different domains, such as finance, healthcare, and manufacturing. However, the inherent difference in the underlying mechanisms of generative AI and traditional AI underscores the unique and complementary roles they play in driving technological innovation and addressing diverse business challenges.
Models and Methods
The foundational models and methods underpinning the capabilities of generative AI are instrumental in shaping the landscape of AI-powered content generation and data synthesis. These sophisticated techniques, which encompass Generative Adversarial Networks (GANs), Variational Autoencoders (VAEs), and Transformers, form the bedrock of generative AI's ability to learn from and generate new content based on intricate patterns and latent structures within the training data. Through the dynamic interplay of these models and methods, generative AI has demonstrated remarkable proficiency in a diverse range of tasks, including natural language processing, image generation, and multi-modal synthesis, thereby unlocking new frontiers in AI-driven content creation and innovation.
Generative Adversarial Networks (GANs)
Generative Adversarial Networks (GANs) represent a pioneering class of generative models that have redefined the landscape of artificial intelligence, particularly in the domain of image generation, data synthesis, and content creation. Comprising of two distinct neural networks, the generator and the discriminator. GANs operate in a competitive and collaborative framework, wherein the generator is tasked with producing synthetic data, while the discriminator evaluates the authenticity of the generated data. This adversarial setting engenders a process of dynamic learning and optimization, ultimately enabling GANs to create high-fidelity and indistinguishable synthetic data, ranging from photorealistic images to simulated real-world scenarios, thereby amplifying the scope and potential of generative AI across diverse domains.
Variational Autoencoders (VAEs)
Variational Autoencoders (VAEs) constitute another foundational architecture within the realm of generative AI, renowned for their proficiency in learning and navigating the latent space of complex data distributions, such as images, text, and audio. By leveraging the principles of probabilistic modeling and variational inference, VAEs empower AI systems to encode and decode high-dimensional data, facilitating the seamless generation of new and diverse content with controllable and structured features. The latent space representation cultivated by VAEs serves as a fundamental substrate for diverse applications, including content synthesis, data interpolation, and anomaly detection, thereby reinforcing the pivotal role of VAEs in the proliferation of generative AI across an array of use cases.
Transformers
Transformers, encompassing seminal architectures like the GPT (Generative Pre-trained Transformer) series, have emerged as a linchpin of generative AI, epitomizing the convergence of large-scale language models and versatile content generation. Fueled by attention mechanisms and self-supervised learning paradigms, Transformers have revolutionized natural language processing and understanding, enabling AI systems to generate coherent and contextually relevant text across diverse genres and domains. Furthermore, the adaptive and expansive nature of Transformers has transcended the confines of language, permeating into the realm of multimodal synthesis and content generation, thereby heralding a new era of AI-driven innovation and creativity.
Applications of Generative AI
The applications of generative AI are as diverse as they are transformative, permeating across an extensive spectrum of domains and industries to engender novel and impactful solutions. Leveraging the inherent capacity of generative AI to create new and authentic content, the technology has catalyzed a paradigm shift in text generation, enabling the seamless synthesis of human-like, contextually relevant, and coherent textual outputs for a myriad of applications. From content generation and curation to creative writing and personalized communication, generative AI has emerged as a potent enabler of linguistic innovation and automation, redefining the contours of human-AI collaboration and interaction in the process.
Text Generation
Generative AI's prowess in text generation has underpinned its pervasive influence across an array of use cases, encompassing natural language understanding, conversational agents, and content generation at an unprecedented scale. By virtue of its proficiency in learning and synthesizing intricate patterns and semantic structures within textual data, generative AI has been instrumental in streamlining content creation, automating workflow processes, and enhancing the overall efficiency and efficacy of linguistic communication. Furthermore, the fusion of generative AI with diverse modalities, such as audio and visual inputs, has precipitated the emergence of multimodal text generation, thereby enriching the breadth and depth of AI-powered content creation and innovation.
Image and Video Synthesis
Beyond text generation, generative AI has wrought a profound impact on the landscape of image and video synthesis, manifesting in the creation of photorealistic images, artistic renderings, and dynamic video content that exemplify the frontiers of AI-enabled visual creativity. Through the dynamic interplay of generative models, including GANs, VAEs, and Transformer-based architectures, AI systems have demonstrated the capacity to engender visually compelling and contextually relevant imagery, ranging from style transfer and artistic synthesis to video inpainting and generative visual storytelling. Consequently, the amalgamation of generative AI with computer vision and visual analytics has fostered a rich tapestry of applications, spanning digital content creation, visual effects, and multimedia production, thereby delineating the transformative and multidimensional impact of generative AI on the creative and visual arts landscape.
Definition and Differences
The essence of generative AI lies in its capacity to usher forth a new paradigm of creativity and innovation, underpinned by the unprecedented ability to learn from and create new data, thereby transcending the bounds of conventional AI paradigms. By harnessing the innate potential of advanced machine learning and deep learning techniques, generative AI has redefined the boundaries of content creation and synthesis, permeating across diverse modalities, including text, images, and videos, to engender a renaissance of AI-enabled creativity and productivity. This fundamental distinction between generative AI and traditional AI underscores the transformative and disruptive potential of the former in reshaping the contours of technological innovation and driving sustainable value across a multiplicity of domains and applications.
The Future of Generative AI
At the vanguard of generative AI's value proposition is its innate capacity to serve as a catalyst for the generation of new and authentic content, thereby redefining the frontiers of creativity, innovation, and productivity. Whether in the domain of art, literature, or business, the deployment of generative AI engenders a seismic shift in the process of content generation and data synthesis, empowering individuals and organizations to harness the full spectrum of AI-driven creativity and ideation. By fostering a symbiotic interplay between human ingenuity and algorithmic creativity, generative AI paves the way for a future replete with novel and compelling content experiences, thereby heralding a new era of AI-facilitated content creation, curation, and dissemination across global digital ecosystems.
As generative AI permeates and proliferates across an expansive array of domains and use cases, from art and literature to business and technology, its transformative potential continues to catalyze a renaissance of creativity, innovation, and productivity. Whether in the guise of GANs conjuring photorealistic artworks, NLP models crafting compelling narratives, or VAEs engendering diverse and dynamic media content, the amalgamation of generative AI with human creativity portrays a synergistic and paradigm-altering fusion that augurs unprecedented opportunities for the co-creation and dissemination of AI-driven content and experiences. The advent of generative AI has not only expanded the frontiers of computational creativity and generativity but has also engendered a transformative and symbiotic ecosystem wherein human ingenuity and AI-enabled creativity converge to redefine the contours of content creation, curation, and consumption at a global scale.
Conclusion
In conclusion, generative AI is a powerful tool that can accelerate application development and enhance business outcomes through the creation of new content. With the help of advanced models and methods, generative AI can generate text, images, and videos, among other data types. Join us at Cream Digital Ai for a free consultation and learn how our AI solutions can boost productivity and efficiency for your business. Book your consultation now and discover the future of AI in business.